Mark III Systems' Introduction to Datasets: Session Three
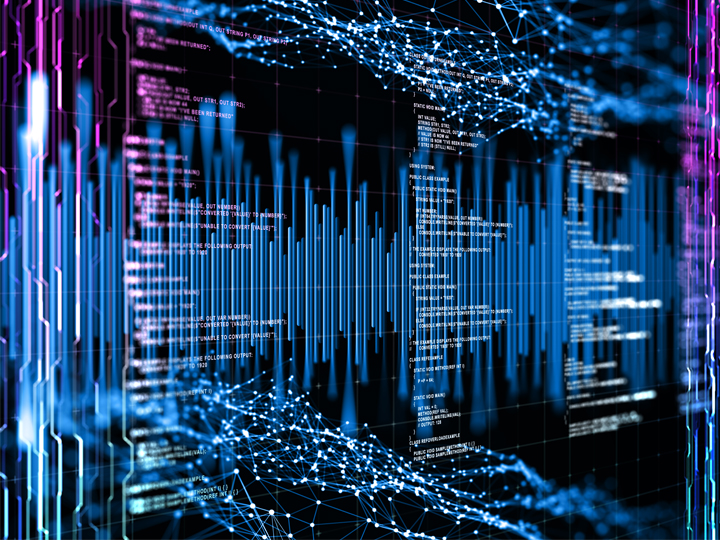
The first piece of advice was to evaluate the problem you are trying to solve – spend as much time on this as any other part of your project. Then decide if you want to use an existing dataset or make your own. There are pre-made datasets from sources such as Keras and Kaggle or you can google your own datasets. There are a good number of search engines that produce usable datasets.
The next step is to look at your dataset and see what changes you have to make to it to use it. According to Buchanan, the consummate data scientist who taught this webinar for Mark III Systems, this takes a “decent bit of work.” Most of the time, you’ll have to make changes/manipulations to any dataset in order to use it.
You may make your own dataset. Things to be aware of if you go this route is to be cognizant of the labeling -- how are you going to make labels for supervised machine learning? You’re also going to need a good variety of images. For instance, if you’re trying to train a model to distinguish cat images from dog images, you can’t have a dataset with thousands of dog images and five cat images.
Kinds of datasets include tabular, computer vision, Natural Language Processing, times series or audio datasets.
Another strategy Buchanan noted was to find a dataset that’s solid, and working backwards from there. She worked on preparing several datasets for training in a Jupyter notebook, simply presenting a complex data problem for those with a background in machine learning and deep learning.